A novel prediction model for the probability of mediastinal lymph node metastases detected by endobronchial ultrasound-transbronchial needle aspiration in non-small cell lung cancer: possible applications in clinical decision-making
Surgery is the most successful radical approach to non-small cell lung cancer (NSCLC), provided accurate preoperative systemic and mediastinal staging for stratification of patients with potentially resectable disease (1). In particular, pre-surgical mediastinal nodal staging (N staging) is performed by a combination of diagnostic tests with different levels of accuracy and invasiveness (2,3), as well as cumulative costs and risks. The optimal diagnostic option is composed by selection of a clinically relevant combination from among computed tomography (CT), positron emission tomography (PET), endobronchial ultrasound-transbronchial needle aspiration (EBUS-TBNA), and endoscopic ultrasound-needle aspiration (EUS-NA) (4). O’Connell and colleagues systematically analysed the factors that should drive the most appropriate diagnostics for pre-surgical N staging and provided a prediction model to help with the assessment of adenopathy in lung cancer (HAL).
A prediction model estimates the probability of an event on the basis of risk factors, thus driving the most appropriate resource in the subpopulation that is likely to get the most value from it (5). Thereby, a prediction model may firstly guide the use of staging procedures, and secondly inform about the likelihood of false negative requiring a further confirmatory test. In the scenario of a known malignancy, like lung cancer, prediction models are particularly useful to reduce harms of over investigation and address the most appropriate therapy (e.g., surgery, radiation therapy, or medical treatment) (6).
Each of CT and PET show limitations in N staging, especially with smaller lymph nodes (7). As a consequence, the American College of Chest Physicians (ACCP) issued guidelines with four main categories on the basis of thoracic radiographic appearance to drive the most appropriate use of EBUS-TBNA according to likelihood of N2/N3 (Table 1), addressing invasive mediastinal staging in case of normal radiographic nodal appearance but relevant PET uptake. A similar approach is replicated by the guidelines from the European Society of Thoracic Surgeons (ESTS) (2)
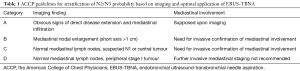
Full table
Yet, those guidelines suffer from the lack of a specific algorithm for risk stratification. Furthermore, it cannot be overemphasised that there is an increasing proportion of lung adenocarcinoma that are not metabolically susceptible under conventional 18-Fluorodeoxyglucose (18FDG) PET (8). The HAL model aims to address such gaps.
The HAL model was developed on retrospective single-centre data, and validated on external cohorts from three further hospitals. The authors call it “parsimonious” because indeed it can be applied by only age and CT data, with further possible integration with PET and tumour histology.
The literature provides a number of models that stratify the risk of N metastases from lung cancer (5,9-17). The major effort of providing specific prediction models turned into the intrinsic limitation of several independent models that heterogeneously covered a variety of specific clinical scenarios (e.g., selection of cT1 lung cancer; Table 2). Most of them focus on stage I neoplasms (9-11,15,16), on a single histologic subtype (10,11), on patients with negative lymph nodes by morphological or metabolic findings (9-11,13,15-17). Also, there is a number of studies that do not include PET for mediastinal stratification (12,13,16,17), despite PET is currently included as standard of care for systemic staging (3). Thereof, compared to former models in the literature (Table 2), the HAL model has the major strength of wide applicability through age decades (e.g., 40–80), NSCLC histology types, and lung cancer stages. The integrity and the wide applicability of a single model through dozens of clinical scenarios make the model a good candidate for inclusion in guidelines. Risk factors retained in the HAL for the probability of N2/N3 (prN2/N3) disease were younger age, adenocarcinoma histology, central location of the tumour (i.e., in the inner one-third of lung parenchyma), and higher N stage demonstrated by PET and CT. Tumour size wasn’t retained in the HAL, in contrast with a previous study from Farjah and colleagues (14).
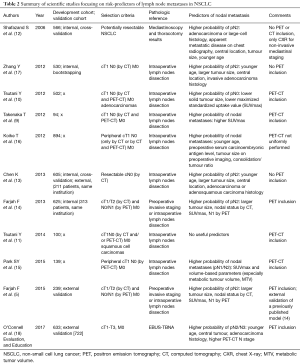
Full table
One could argue that prediction models might perform differently according to several clinical variables that might change between individual hospitals. The external validation is the method of testing the broad applicability of a proposed model (6). The previously proposed models almost entirely missed external validation, with the exception of the study from Farjah et al. (5). It is interesting that indeed the first version of the HAL model was found overestimating prN2/N3 in the three external validation cohorts. Of note, the technique for EBUS-TBNA was consistent between the developing and validation cohort: sampling of each lymph node >0.5 cm (e.g., minimized likelihood of false negative). In this regard, the external validation operated by O’Connell et al. clarifies the adjustment needed for its universal application. Noteworthy, this model accounts for inter-centre variability, and a method of calibration was proposed to grant consistent performance of the model over a range of different local patient patterns. This latter feature is particularly convenient in clinical practice where accuracy and reproducibility are quite debated at the level of the diagnostic test. Indeed, the calibration proposed by O’Connell comes with an upper hierarchical degree that aims to minimize differences in the final outcome: prN2/N3 by EBUS-TBNA.
A potential limitation of the study by O’Connell lays in the use of EBUS-TBNA as standard of reference for nodal status assessment, rather than thoracotomy. In fact, lymph node sampling by EBUS-TBNA could underestimate the real prevalence of disease: the ACCP guidelines report a sensitivity 89% and specificity 100%. However, it should be noted that such 10% variability in sensitivity is still acceptable, as long as specificity is perfect. Indeed, the ESTS guidelines (2) deem acceptable up to 10% of unforeseen pathologic N2 patients (mainly represented by single N2 stations) revealed by surgical resection despite preoperative accurate staging. Hence, as advocated by O’Connell and colleagues, a second and relevant possible application of the aforementioned model is to estimate the probability of a false negative EBUS-TBNA result with the attempt to determine whether a confirmatory mediastinoscopy should be considered.
Secondary purpose of the study by O’Connell and colleagues was to investigate the role of PET in the diagnostic algorithm, after a chest CT scan. The model showed that, in case of obvious N2 disease at CT, the clinical yield of PET imaging is limited to detection of distant metastases. Apart from systemic metastases, CT findings combined to clinical data would consistently indicate appropriateness of EBUS-TBNA, irrespective of PET results. On the other hand, in N0 patients by CT, the HAL underscores the additional value of PET imaging.
Furthermore, three additional multivariable models are provided by the authors to inform decision making in different clinical scenarios, demonstrating good similar performance of the prediction rule also when both PET and histology are not known. In particular, the area under the receiving operator curve (AUC) was 0.88 for the full model including histology characterization. Nonetheless, the AUC for a restricted model without histology still performed well (AUC 0.87), suggesting that the HAL might be used even before biopsy of the primary lung cancer. On the other hand, the detrimental effect of pre-PET and pre-biopsy application of the model seems to be more conspicuous (AUC 0.76) and might lead to a greater level of uncertainty between predicted and observed nodal N2/N3 involvement. Further studies are warranted to test the performance of such model for alternative preoperative diagnostic algorithms.
Finally, it seems that there might be some degree of association between the prN2/N3 and the risk of false negatives at EBUS-TBNA. As clearly demonstrated, the main goal of the HAL model is to determine whether EBUS-TBNA is recommended in different clinical settings, advising a 10% threshold of predicted N2/N3 involvement (Figures 1-3). Moreover, the model shows that negative EBUS-TBNA might be found with a likelihood of actual N2/N3 positivity still above the 10% (Figure 4). Should this be an indication to proceed directly to mediastinoscopy in such high-risk patients, and maybe use the HAL to leave the EBUS-TBNA to cases with more limited risk of false negative? Furthermore, it should be remembered that the ESTS guidelines (2) generally recommend a further confirmatory invasive investigation (i.e., video-assisted mediastinoscopy) in patients suspected for mediastinal involvement by PET-CT but negative after EBUS-TBNA. The possibility of a future integration of a prediction model in the guidelines follows the importance of estimating the pre-test and post-test probability of nodal metastases with the aim to minimize the number of unnecessary invasive procedures performed. The HAL model is made available for further validation, we foster the literature will provide more evidence and critical testing of this promising novel comprehensive predictor of mediastinal involvement in lung cancer.
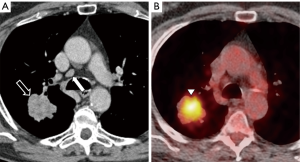
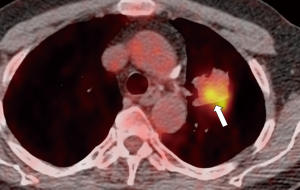
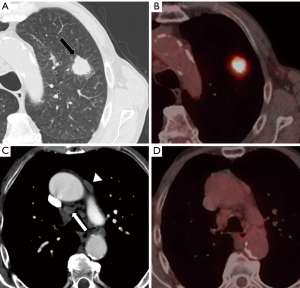
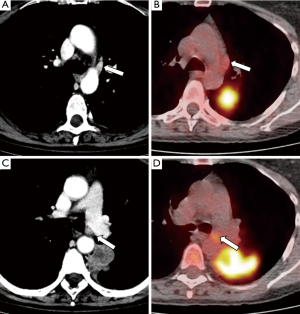
In conclusion, prediction models may be considered a useful tool to guide decision-making for patients with potentially resectable lung cancer, with the aim of reducing the number of invasive mediastinal staging procedures. The HAL model seems to have the potential for medical decision support to guide invasive mediastinal staging, also warranting a further confirmatory investigation (i.e., mediastinoscopy) after a first EBUS-TBNA result suspicious for false-negative.
Acknowledgments
Funding: None.
Footnote
Provenance and Peer Review: This article was commissioned and reviewed by the Section Editor Dr. Luigi Ventura (Thoracic Surgery, Surgical Unit, Department of Medicine and Surgery, University Hospital of Parma, Parma, Italy).
Conflicts of Interest: All authors have completed the ICMJE uniform disclosure form (available at http://dx.doi.org/10.21037/med.2018.05.02). The authors have no conflicts of interest to declare.
Ethical Statement: The authors are accountable for all aspects of the work in ensuring that questions related to the accuracy or integrity of any part of the work are appropriately investigated and resolved.
Open Access Statement: This is an Open Access article distributed in accordance with the Creative Commons Attribution-NonCommercial-NoDerivs 4.0 International License (CC BY-NC-ND 4.0), which permits the non-commercial replication and distribution of the article with the strict proviso that no changes or edits are made and the original work is properly cited (including links to both the formal publication through the relevant DOI and the license). See: https://creativecommons.org/licenses/by-nc-nd/4.0/.
References
- Rosen JE, Keshava HB, Yao X, et al. The Natural History of Operable Non-Small Cell Lung Cancer in the National Cancer Database. Ann Thorac Surg 2016;101:1850-5. [Crossref] [PubMed]
- De Leyn P, Dooms C, Kuzdzal J, et al. Revised ESTS guidelines for preoperative mediastinal lymph node staging for non-small-cell lung cancer. Eur J Cardiothorac Surg 2014;45:787-98. [Crossref] [PubMed]
- Shen KR, Meyers BF, Larner JM, et al. Special Treatment Issues in Lung Cancer* ACCP Evidence-Based Clinical Practice Guidelines (2nd Edition). Chest 2007;132: 290S-305S.
- Goldstraw P, Chansky K, Crowley J, et al. The IASLC Lung Cancer Staging Project: Proposals for Revision of the TNM Stage Groupings in the Forthcoming (Eighth) Edition of the TNM Classification for Lung Cancer. J Thorac Oncol 2015;11:39-51. [Crossref] [PubMed]
- Farjah F, Backhus LM, Varghese TK, et al. External validation of a prediction model for pathologic N2 among patients with a negative mediastinum by positron emission tomography. J Thorac Dis 2015;7:576-84. [PubMed]
- Steyerberg EW, Vickers AJ, Cook NR, et al. Assessing the performance of prediction models: a framework for traditional and novel measures. Epidemiology 2010;21:128-38. [Crossref] [PubMed]
- Nambu A, Kato S, Motosugi U, et al. Thin-section CT of the mediastinum in preoperative N-staging of non-small cell lung cancer: comparison with FDG PET. Eur J Radiol 2010;73:510-7. [Crossref] [PubMed]
- Tsubakimoto M, Yamashiro T, Tamashiro Y, et al. Quantitative CT density histogram values and standardized uptake values of FDG-PET/CT with respiratory gating can distinguish solid adenocarcinomas from squamous cell carcinomas of the lung. Eur J Radiol 2018;100:108-15. [Crossref] [PubMed]
- Takenaka T, Yano T, Morodomi Y, et al. Prediction of true-negative lymph node metastasis in clinical IA non-small cell lung cancer by measuring standardized uptake values on positron emission tomography. Surg Today 2012;42:934-9. [Crossref] [PubMed]
- Tsutani Y, Miyata Y, Nakayama H, et al. Prediction of pathologic node-negative clinical stage IA lung adenocarcinoma for optimal candidates undergoing sublobar resection. J Thorac Cardiovasc Surg 2012;144:1365-71. [Crossref] [PubMed]
- Tsutani Y, Murakami S, Miyata Y, et al. Prediction of lymph node status in clinical stage IA squamous cell carcinoma of the lung. Eur J Cardiothorac Surg 2015;47:1022-6. [Crossref] [PubMed]
- Shafazand S, Gould MK. A clinical prediction rule to estimate the probability of mediastinal metastasis in patients with non-small cell lung cancer. J Thorac Oncol 2006;1:953-9. [Crossref] [PubMed]
- Chen K, Yang F, Jiang G, et al. Development and validation of a clinical prediction model for N2 lymph node metastasis in non-small cell lung cancer. Ann Thorac Surg 2013;96:1761-8. [Crossref] [PubMed]
- Farjah F, Lou F, Sima C, et al. A prediction model for pathologic N2 disease in lung cancer patients with a negative mediastinum by positron emission tomography. J Thorac Oncol 2013;8:1170-80. [Crossref] [PubMed]
- Park SY, Yoon JK, Park KJ, et al. Prediction of occult lymph node metastasis using volume-based PET parameters in small-sized peripheral non-small cell lung cancer. Cancer Imaging 2015;15:21. [Crossref] [PubMed]
- Koike T, Koike T, Yamato Y, et al. Predictive risk factors for mediastinal lymph node metastasis in clinical stage IA non-small-cell lung cancer patients. J Thorac Oncol 2012;7:1246-51. [Crossref] [PubMed]
- Zhang Y, Sun Y, Xiang J, et al. A prediction model for N2 disease in T1 non-small cell lung cancer. J Thorac Cardiovasc Surg 2012;144:1360-4. [Crossref] [PubMed]
- O’Connell OJ, Almeida FA, Simoff MJ, et al. A Prediction Model to Help with the Assessment of Adenopathy in Lung Cancer: HAL. Am J Respir Crit Care Med 2017;195:1651-60. [Crossref] [PubMed]
Cite this article as: Carnevale A, Milanese G, Sverzellati N, Silva M. A novel prediction model for the probability of mediastinal lymph node metastases detected by endobronchial ultrasound-transbronchial needle aspiration in non-small cell lung cancer: possible applications in clinical decision-making. Mediastinum 2018;2:43.